Home / Intelligence / Blog / The Future of AI for Market Access: An ISPOR US 2023 Recap
Published July 17, 2023
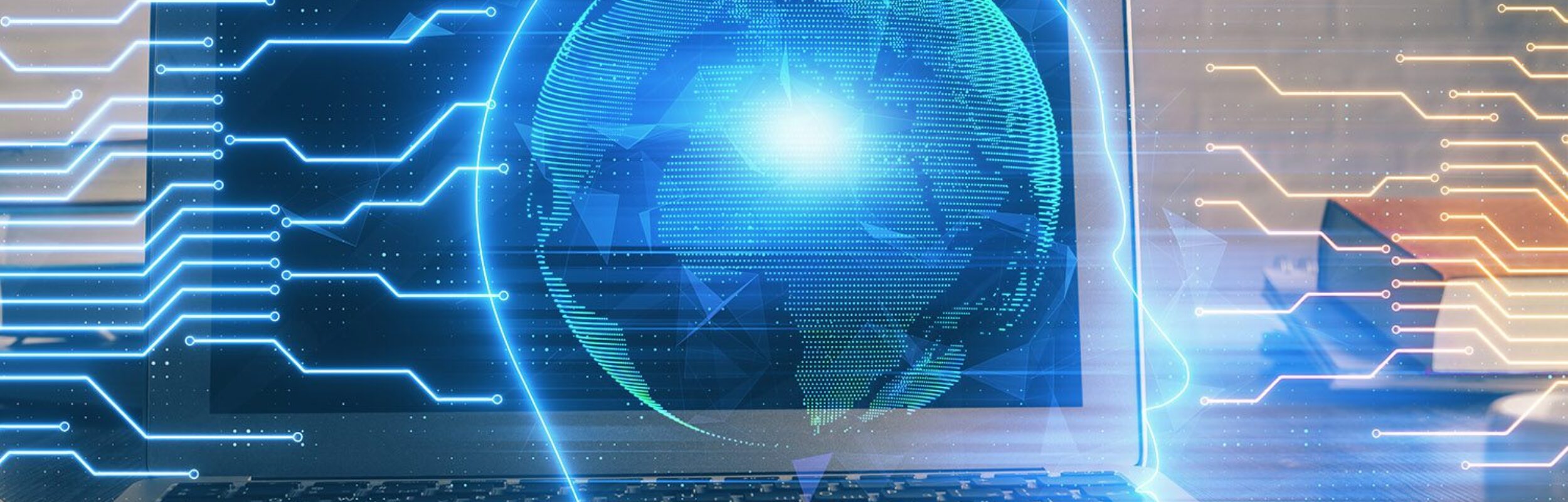
This year Trinity team members at ISPOR 2023 attended several symposia and presentations on the use of Artificial Intelligence (AI) to support biopharma evidence generation. Among many industry trends, experts anticipate that AI and machine learning will increasingly be leveraged to identify optimal patient populations and expedite the path toward personalized medicine.
Pioneering New Frontiers in Health Economics and Market Access: The transformative power of AI, ML and advanced data analytics
This symposium focused on the role of innovative technologies in healthcare and their potential impact on market access. With recent innovations in CGT, immuno-oncology and RNA vaccines, and the increasing presence of combination approaches and personalized medicine, the landscape of evidence generation is becoming more complex. Presenters discussed how these technologies can help establish an integrated evidence plan earlier in the asset lifecycle than ever before.
As most products coming to market offer incremental added benefit compared to existing options, demonstrating value for access will more and more rely on real-world data to optimize reimbursement potential. To add complexity, products must demonstrate value to different stakeholders in different ways: efficacy to regulators, cost-effectiveness to payers and humanistic values to patients.
Artificial intelligence enables researchers to manage large data sets, identify patterns and mimic decision-making processes to inform business decisions. Panelists highlighted the potential for AI to directly supplement HEOR analyses, like automating systematic literature reviews, but experts still posed the questions: “If AI can understand payer and patient behaviors, the landscape and available therapies, how can we leverage it to inform successful HTA evaluations?”; “Will payers accept AI-generated indirect treatment comparison (ITC) data to increase precision and robustness?”, highlighting the uncertainty that still exists in this field.
Use of AI to develop Health Economic evidence for optimized patient access
AI technologies have the potential to transform evidence generation by leveraging electronic health records, claims data, health technology assessments, regulatory assessments and patient-generated data. AI can derive new insights from these rich data sources, identify patient subpopulations, understand disease burdens and enhance treatment strategies. Panelists emphasized how AI and machine learning can improve access to critical information for patients, clinicians, payers and stakeholders, leading to better-informed decision-making, efficient resource utilization and improved patient care.
However, there are potential concerns with data privacy and security as effective use of AI models requires access to detailed and large datasets. Moreover, researchers noted potential biases that could be introduced into AI-based analyses that could overlook trends for certain patient groups. Ultimately, market access and HEOR experts must balance AI-driven efficiency with ethical concerns when integrating these new technologies into drug access frameworks.
AI Wants to Chat With You: Accept or Ignore?
As AI becomes more broadly accepted in healthcare, experts expect it will be a tool to help generate RWE to inform regulatory approval, but not replace the provider. Large language models (LLMs) can classify unstructured data with the oversight of clinicians who can understand the data. For example, AI is already being tested to map patient conversations to validated survey instruments to alleviate patient fatigue due to repeating their condition and increase response rates. AI can enable patient voice through the quantification of disease burden while expediating chart review and physician burden.
The main concern, which prevails in all applications of AI, is the implicit bias associated with models. Though these tools allow HCPs to engage the patient more directly, they must also be tested through a health equity lens to mitigate potential disparities that may be implicit in source data.
Conclusion
The integration of AI, ML and advanced data analytics in health economics and market access offers immense potential for transforming healthcare. By adopting an integrated evidence plan early in the asset lifecycle, generating health economic evidence, optimizing patient access and addressing ethical considerations, AI becomes an invaluable tool for healthcare decision-making. However, careful attention must be given to ethical concerns, bias mitigation and the balanced application of AI to ensure fair and equitable outcomes in health economics and market access.
Authors: Max Hunt, Ismail Ismailoglu, Grace Mock, Cameron Lam and Alexis Bryan
Related Intelligence
Webinars
Virtual Demo | HTA Vision with GenAI
Available On Demand
Join Trinity Life Sciences for a virtual demo where value, access and pricing experts Max Hunt and Andreia Ribeiro will demonstrate Trinity HTA Vision, our cloud-based dashboard with GenAI capabilities. It is not enough to know what the health technology assessment (HTA) decisions are and when they are made—with the landscape continually evolving, analogues and future […]
Watch Now
Blog
Learnings to Kick-Start 2025: Health Equity
Health equity continues to be a hot topic in the life sciences industry. ISPOR EU sessions addressed several key questions surrounding the impact of health equity on HTA decision-making, the methodologies that should be employed, and the opportunities and challenges of incorporating health equity into health technology assessment (HTA). Ahead of ISPOR EU, Trinity’s HTA […]
Read More
Blog
Maximum Fair Price Round 2: Passing the Baton
On January 20, 2025, CMS announced the set of fifteen drugs targeted for the second round of Maximum Fair Price (MFP) “negotiations” under IRA. With the outcomes of the first price “negotiations” announced only last August, the IRA MFP process is still in its early development and there remains uncertainty over how it will evolve […]
Read More